|
ACCESS THE FULL ARTICLE
No SPIE Account? Create one
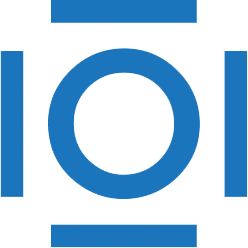
CITATIONS
Cited by 51 scholarly publications.
Hyperspectral imaging
Image classification
Image segmentation
Databases
Classification systems
Feature extraction
Visualization