Representation-based classification methods (RBCM) remain one of the hottest topics in the community of pattern recognition, and the recently proposed non-negative representation-based classification (NRC) achieved impressive recognition results in various classification tasks. However, NRC ignores the relationship between the coding and classification stages. Moreover, there is no regularization term other than the reconstruction error term in the formulation of NRC, which may result in an unstable solution leading to misclassification. To overcome these drawbacks of NRC, we propose a class-specific residual constraint non-negative representation (CRNR) for pattern classification. CRNR introduces a class-specific residual constraint into the formulation of NRC, which encourages training samples from different classes to competitively represent the test sample. Based on the proposed CRNR, we develop a CRNR-based classifier (CRNRC) for pattern classification. Experimental results on several benchmark datasets demonstrate the superiority of CRNRC over conventional RBCM as well as the recently proposed NRC. Moreover, CRNRC works better than or comparable to some state-of-the-art deep approaches on diverse challenging pattern classification tasks. |
ACCESS THE FULL ARTICLE
No SPIE Account? Create one
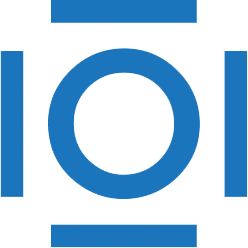
CITATIONS
Cited by 4 scholarly publications.
Image classification
Databases
Error control coding
Associative arrays
Autoregressive models
Facial recognition systems
Chromium