Small object detection is always a hot research direction in the field of computer vision, and widely used in traffic, production, industry, and other fields. A neural network for detecting small objects based on original Cascade RCNN is proposed. First, we modify the traditional feature pyramid network and introduce multi-branch dilated convolutions to enhance the feature information of the small target. Second, the feature extraction networks in original Cascade RCNN are replaced with multi-layer deformable convolution networks, which can better adapt to the geometric variations of detection target and huge size span of the objects in the same scene. Finally, Soft non-maximum suppression is also integrated into the network to avoid problems in dense object detection. To verify the practicability of our proposed network, we apply it to the garbage detection system. The experimental results show that compared with original Cascade RCNN and other commonly used target detection networks, our proposed method, with a recall rate >0.98 as well as mean average precision up to 0.964, performs better not only in small object detection but also in industrial applications. |
ACCESS THE FULL ARTICLE
No SPIE Account? Create one
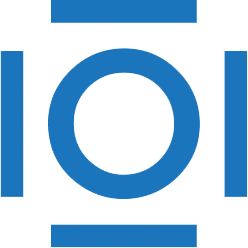
CITATIONS
Cited by 5 scholarly publications.
Convolution
Target detection
Feature extraction
Intelligence systems
Sensors
Detection and tracking algorithms
Imaging systems