Convolutional neural networks (CNNs) are effective for image classification, and deeper CNNs are being used to improve classification performance. Indeed, as needs increase for searchability of vast printed document image collections, powerful CNNs have been used in place of conventional image processing. However, better performances of deep CNNs come at the expense of computational complexity. Are the additional training efforts required by deeper CNNs worth the improvement in performance? Or could a shallow CNN coupled with conventional image processing (e.g., binarization and consolidation) outperform deeper CNN-based solutions? We investigate performance gaps among shallow (LeNet-5, -7, and -9), deep (ResNet-18), and very deep (ResNet-152, MobileNetV2, and EfficientNet) CNNs for noisy printed document images, e.g., historical newspapers and document images in the RVL-CDIP repository. Our investigation considers two different classification tasks: (1) identifying poems in historical newspapers and (2) classifying 16 document types in document images. Empirical results show that a shallow CNN coupled with computationally inexpensive preprocessing can have a robust response with significantly reduced training samples; deep CNNs coupled with preprocessing can outperform very deep CNNs effectively and efficiently; and aggressive preprocessing is not helpful as it could remove potentially useful information in document images. |
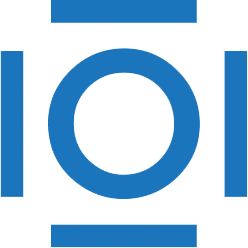
CITATIONS
Cited by 2 scholarly publications and 1 patent.
Image classification
Visualization
Performance modeling
Binary data
Image processing
Image filtering
Convolutional neural networks