There is a dire need for vision automation for edible bird’s nest (EBN) hygiene inspection. To date, an effective impurities detection method for EBNs has yet to be realized owing to the inhomogeneous optical properties, various types and sizes of impurities, and limited sample size. The impurities inspection was formulated as an anomaly detection task, and a hybrid autoencoder model that contains an autoencoder and a single layer convolutional network is proposed. The model was trained to reconstruct only nonimpurity regions of the EBN for impurities segmentation and detection as anomalies. The results showed that with only 50 EBN sample images, the hybrid model achieved a recall of 0.9282, a precision of 0.7718, and a 5.63% undetected rate for impurities. Furthermore, a misclassification rate of 21.53% was recorded due to artifacts mostly with sizes <0.20 mm that were detected as false positive. Nonetheless, the applicability of the proposed autoencoder model was confirmed, with >92 % of successful impurities detected. Therefore, the hybrid autoencoder model is further explored for improvement and practical application. |
ACCESS THE FULL ARTICLE
No SPIE Account? Create one
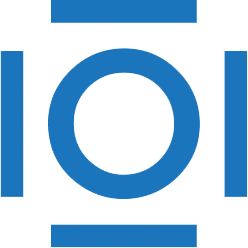
CITATIONS
Cited by 2 scholarly publications.
Image segmentation
Inspection
Performance modeling
Visual process modeling
Image processing
Statistical modeling
Optical properties