Purpose: Voxel-level hypothesis testing on images suffers from test multiplicity. Numerous correction methods exist, mainly applied and evaluated on neuroimaging and synthetic datasets. However, newly developed approaches like Imiomics, using different data and less common analysis types, also require multiplicity correction for more reliable inference. To handle the multiple comparisons in Imiomics, we aim to evaluate correction methods on whole-body MRI and correlation analyses, and to develop techniques specifically suited for the given analyses. Approach: We evaluate the most common familywise error rate (FWER) limiting procedures on whole-body correlation analyses via standard (synthetic no-activation) nominal error rate estimation as well as smaller prior-knowledge based stringency analysis. Their performance is compared to our anatomy-based method extensions. Results: Results show that nonparametric methods behave better for the given analyses. The proposed prior-knowledge based evaluation shows that the devised extensions including anatomical priors can achieve the same power while keeping the FWER closer to the desired rate. Conclusions: Permutation-based approaches perform adequately and can be used within Imiomics. They can be improved by including information on image structure. We expect such method extensions to become even more relevant with new applications and larger datasets. |
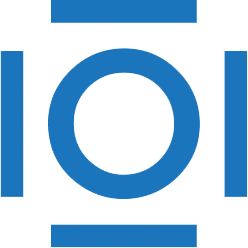
CITATIONS
Cited by 4 scholarly publications.
Magnetic resonance imaging
Image segmentation
Tissues
Error analysis
Neuroimaging
Statistical analysis
Visualization