Purpose: The coronary arteries are embedded in a layer of fat known as epicardial adipose tissue (EAT). The EAT influences the development of coronary artery disease (CAD), and increased EAT volume can be indicative of the presence and type of CAD. Identification of EAT using echocardiography is challenging and only sometimes feasible on the free wall of the right ventricle. We investigated the use of spectral analysis of the ultrasound radiofrequency (RF) backscatter for its potential to provide a more complete characterization of the EAT. Approach: Autoregressive (AR) models facilitated analysis of the short-time signals and allowed tuning of the optimal order of the spectral estimation process. The spectra were normalized using a reference phantom and spectral features were computed from both normalized and non-normalized data. The features were used to train random forests for classification of EAT, myocardium, and blood. Results: Using an AR order of 15 with the normalized data, a Monte Carlo cross validation yielded accuracies of 87.9% for EAT, 84.8% for myocardium, and 93.3% for blood in a database of 805 regions-of-interest. Youden’s index, the sum of sensitivity, and specificity minus 1 were 0.799, 0.755, and 0.933, respectively. Conclusions: We demonstrated that spectral analysis of the raw RF signals may facilitate identification of the EAT when it may not otherwise be visible in traditional B-mode images. |
ACCESS THE FULL ARTICLE
No SPIE Account? Create one
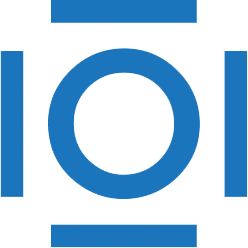
CITATIONS
Cited by 1 scholarly publication.
Autoregressive models
Tissues
Ultrasonography
Backscatter
Blood
Data modeling
Signal processing