ACCESS THE FULL ARTICLE
No SPIE Account? Create one
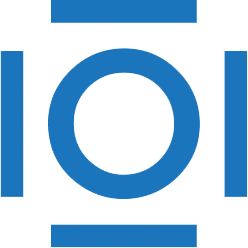
CITATIONS
Cited by 2 scholarly publications.
Tumors
Image segmentation
Computed tomography
Lung cancer
Positron emission tomography
Bone
Data modeling