Background: Deep learning is a very fast-growing field in the area of artificial intelligence with remarkable results in recent years. Many works in the lithography and photomask field have shown progress of technology in this application area and the potential to improve lithography by the automation of processes. Despite this progress, the use of machine learning techniques in the field of mask repair still seems to be at the beginning. Aim: We show that deep learning-based methods can successfully be applied to mask repair applications. Approach: The presented system is a hybrid and modular approach based on a combination of several deep learning networks and analytical methods, enabling the detection of mask pattern defects and the determination of the exact defect shapes from SEM images. In the current version, the system is trained for line/space patterns and contact patterns with typical defect types. The modularity allows for the extensibility to new use cases. The issue of an insufficient amount of training data is addressed using purely computer-generated simplified SEM data in combination with a specific network architecture. Results: The very good functionality and defect detection accuracy of the system are demonstrated with a set of real SEM images with line/space patterns and contact patterns with numerous defects. In particular, a 100% true defect detection rate could be obtained. Conclusions: The presented machine learning approach demonstrates the successful defect identification, location, and shape determination from real mask SEM images. |
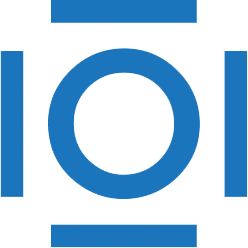
CITATIONS
Cited by 2 scholarly publications.
Scanning electron microscopy
Defect detection
Photomasks
Image segmentation
Image processing
Lithography
Network architectures