|
ACCESS THE FULL ARTICLE
No SPIE Account? Create one
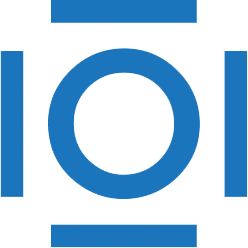
CITATIONS
Cited by 1 scholarly publication.
Calibration
Cameras
Neural networks
Neurons
3D modeling
Artificial neural networks
Optical sensors