|
ACCESS THE FULL ARTICLE
No SPIE Account? Create one
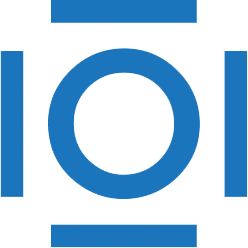
CITATIONS
Cited by 1 scholarly publication.
Statistical modeling
Data modeling
Video
Visualization
Optical engineering
Performance modeling
Machine learning