|
ACCESS THE FULL ARTICLE
No SPIE Account? Create one
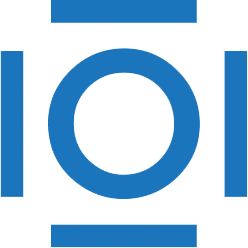
CITATIONS
Cited by 1 scholarly publication.
Image segmentation
Fuzzy logic
Optical microscopy
Brain
Neuroimaging
Magnetorheological finishing
Optical engineering