|
ACCESS THE FULL ARTICLE
No SPIE Account? Create one
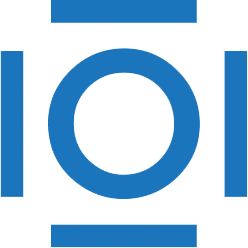
CITATIONS
Cited by 9 scholarly publications.
Compressed sensing
Gallium nitride
Data modeling
Image resolution
Reconstruction algorithms
Image processing
Matrices