Structured light profilometry (SLP) is now widely utilized in noncontact three-dimensional (3D) reconstruction due to its convenience in dynamic measurements. Compared with classic fringe projection profilometries, multiple deep neural networks are proposed to demodulate or unwrap the fringe phase, and these networks utilize convolution layers to extract local features while omitting global characteristics. In this paper, we propose SwinConvUNet, a deep neural network for single-shot SLP that can extract local and global features simultaneously. In the network structure design, convolution layers are applied in shallow layers to extract local features, whereas transformer layers extract global features in deep layers, and an improved loss function by combining gradient-based structural similarity is employed to improve reconstruction details. The experimental results demonstrate that SwinConvUNet is more effective than the U-net model at decreasing learnable parameters while maintaining 3D reconstruction accuracy. |
ACCESS THE FULL ARTICLE
No SPIE Account? Create one
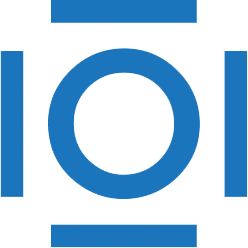
CITATIONS
Cited by 1 scholarly publication.
Convolution
3D modeling
Structured light
Transformers
Fringe analysis
Optical engineering
3D metrology