|
ACCESS THE FULL ARTICLE
No SPIE Account? Create one
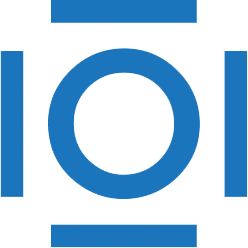
CITATIONS
Cited by 2 scholarly publications.
Positron emission tomography
Computed tomography
Computer programming
Diagnostics
Image quality
Image enhancement
Network architectures