|
ACCESS THE FULL ARTICLE
No SPIE Account? Create one
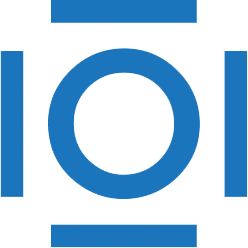
CITATIONS
Cited by 3 scholarly publications.
Filtering (signal processing)
Interference (communication)
Data fusion
Principal component analysis
Error analysis
Signal processing
Global Positioning System