|
ACCESS THE FULL ARTICLE
No SPIE Account? Create one
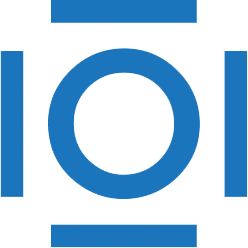
CITATIONS
Cited by 4 scholarly publications.
Breast
Image segmentation
Tissues
Magnesium
Mammary gland
Computed tomography
Breast cancer