|
ACCESS THE FULL ARTICLE
No SPIE Account? Create one
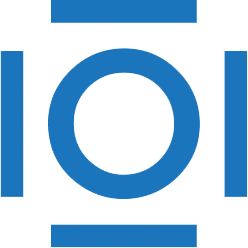
CITATIONS
Cited by 4 scholarly publications.
Machine learning
Data modeling
Genetic algorithms
Situational awareness sensors
Error analysis
Statistical analysis
Classification systems