1.IntroductionSince its first application to study infant development (0 to 2 years),1 functional near-infrared spectroscopy (fNIRS) has rapidly become a highly popular method for developmental neuroimaging. It is used particularly frequently to image the brain of very young individuals, as the relatively thin skull and tissues of infants and toddlers allow light to penetrate deeper into the cerebral cortex than in older populations. Furthermore, other imaging methods, such as functional magnetic resonance imaging (fMRI), are often too constraining or invasive to be used with infants and young children. fNIRS is also easy to use and relatively tolerant to motion. It is thus a particularly suitable methodological choice for developmental populations. These advantages notwithstanding, infants, and toddlers are challenging research participants who have a short attention span, do not understand and/or necessarily comply with instructions, do not easily stay still or may be unwilling to accept the fNIRS cap. In the two and a half decades, since its first use with infants, fNIRS technology, experimental methods, and data analysis techniques have evolved considerably2–4 to meet the special needs and challenges of developmental neuroimaging. As a result, developmental fNIRS research is growing exponentially5 (Fig. 1). Indeed, since 2010, growth has been particularly fast, possibly related to increasing fNIRS expertise in the developmental community as well as the appearance of a greater variety of commercially available systems in more accessible price ranges. Relative to fMRI and electroencephalography (EEG), since 2017, the distribution of new infant development publications (with 0- to 2-year-old) showed a higher increase in fNIRS suggesting a potential shift in the choice of methods for infant studies.6 Fig. 1Increase in number of published infant studies (with 0- to 2-year-old) using fNIRS. Since its inception as an infant neuroimaging technique, fNIRS has been used in an increasing number of infant cognitive neuroscience experiments. Figure adapted with permission from Ref. 5. ![]() In this paper, we consider some of the most recent advances in developmental fNIRS and outline the challenges and perspectives that developmental fNIRS research is likely to face in the near future. Our aim is not to provide a review of the existing developmental fNIRS literature, but rather to highlight current trends and challenges that we believe will shape the future of the developmental fNIRS field. 1.1.Previous Perspectives on Developmental fNIRSAlthough fNIRS had been applied to developmental research soon after its first appearance, early studies were mainly confirmatory, seeking convergence with existing empirical results established through other imaging and behavioral methods and establishing regional selectivity/localization for certain cognitive or perceptual functions (for reviews, see Refs. 2, 3, 7, and 8). Indeed, a 2012 review paper4 states that “…[t]he most important question one can ask about [f]NIRS findings from infants is: What have we learned that we did not know already from other measures? That is, what is the “value-added” of [f]NIRS for studies of infant perception, cognition, and language?.” This is a key question and one that certainly has not left the field. A number of the new directions emerging in the field of developmental fNIRS are motivated by exactly these questions. Here we will consider a number of exciting new directions through this lens: how are they value-added? We take value-added to mean studies that reveal more about the topic of interest (e.g., a clinical population, underlying cognitive mechanisms, and developmental changes) than what could be gleaned without using fNIRS. The previous review4 also raises the issue of replicability, and how the developmental fNIRS field can move toward more hypothesis-driven research rather than exploratory. A decade ago, another challenge facing the field was conducting studies across multiple age groups cross-sectionally and, whenever possible, longitudinally. Beyond the usual practical and methodological difficulties of conducting studies with multiple age groups, this raises a particular challenge for fNIRS. For example, there are developmental changes in neurovascular coupling, an issue that remains poorly understood even today,9,10 and changes in the neuroanatomy of the brain which make localization across ages a challenge.11,12 The increasing technological advances of NIRS technology and, neuroimaging in general, have also brought about new challenges and opportunities. There is an ever-stronger impetus for research with human participants to be inclusive, investigating the perceptual, cognitive, affective, and neural abilities of individuals who rarely participated in laboratory studies before. This has led to developmental fNIRS research being taken outside the laboratory to reach global populations, to test participants in their natural environments, and to assess individual variation. These advances also require increasingly complex and sophisticated methodological procedures and data analysis techniques, many of which are being adapted to fNIRS from other imaging modalities and computational approaches, such as resting state and functional connectivity analysis or machine learning. The field is currently making considerable efforts to address the issues outlined above, as overviewed in the following sections. 1.2.Current PaperWe review the most recent challenges and opportunities in developmental fNIRS with the aim of providing insights into the trends that will likely shape the future of the field. Specifically, we present the technological advances, for instance, hyperscanning and wearable systems, and the applications that have been brought about by the growing need to conduct more inclusive and more ecologically valid research, taking fNIRS out of laboratories, “into the wild” and across the globe. We discuss the increasing attention paid to individual variation and developmental trajectories, including longitudinal research spanning several time points, sometimes across a number of years. We also review the data processing and analysis techniques that have accompanied these advances as well as the latest initiatives aiming to guarantee the reliability and reproducibility of fNIRS results. 2.Bringing Developmental Neuroimaging into More Ecologically Valid, Diverse, and Naturalistic ContextsAlthough research within a laboratory setting has provided great advances in our understanding of human development, extending the ecological validity of these results is of increasing importance. Research in laboratories is well-suited to some developmental phenomena. Indeed, many of young infants’ earliest abilities have been revealed in controlled research environments (i.e., video-screen-based stimuli with strict parameters to account for perceptual auditory and visual differences), using NIRS (for reviews, see Refs. 2, 3, 7, and 8), and this will continue to be an appropriate design for many theoretically motivated questions even in the future. Several of these lab-based studies have taken advantage of the flexibility of fNIRS to investigate questions using more realistic, ecologically valid stimuli (e.g., puppet theater to test object perception,13 live presentation of goal directed actions,14 and live interactions between researcher and infants to measure ostensive signals15). Indeed, one of the earliest fNIRS studies with infants combined a naturalistic, live researcher–infant interaction paradigm (A-not-B task) with a longitudinal design to investigate the neural underpinnings of this behavioral phenomenon in infancy.16 However, other phenomena, e.g., those that are inherently multimodal, multidimensional, or dynamic, may only be partially explored in the restricted context of a laboratory, impeding our full understanding of the research questions posed. For instance, in social cognition, a central theme in developmental psychology and an area of exponential growth in infant fNIRS,5 infants’ social skills have classically been assessed using static pictures or video stimuli of social signals to better control the experimental contrasts under investigation. However, research has shown the critical role of live social stimuli in infants,17 as exemplified by the phenomenon “video deficit,” whereby infants tend to show difficulties in learning or performing via unidirectional, nonlive video stimuli/presentations. For example, live “peek-a-boo” elicited more prefrontal responses in infants than nonlive presentations18,19 and live action observations produced adult-like activation patterns in motor areas while a televised version did not.14 These differences can be explained by factors, such as perceptual saliency (e.g., 2D versus 3D), bidirectionality, and contingent responsiveness,20 and highlight the importance of embedding ecologically valid designs in our research program to better study context-sensitive, multidimensional, or dynamic phenomena. Research in labs can also result in highly biased sociocultural sampling. For example, research labs are predominantly located in universities or hospitals in cities and therefore recruit urban and suburban populations. Furthermore, socially, culturally, or financially disadvantaged groups—those most at risk for experiencing socioeconomic and health challenges—are far less likely to participate in research.6,21 To include previously underrepresented ethnic-racial and geographical groups in developmental research,22 we must seek to reach out beyond lab-based settings. In recent years, the portability of fNIRS has allowed researchers to use more naturalistic contexts and to bring neuroimaging technologies to populations not historically included in developmental research.23 For example, fNIRS was recently applied in a rural context in Côte d’Ivoire, where the instrumentation was transported village to village in a jeep.24 Moreover, fNIRS technology and technological innovation is more commonly taking place in lower-resource research labs that formerly did not have access to such technology, such as the use of high-density optical imaging in a cohort of 7- to 10-year-old children in Colombia.25 Below, we highlight two particular technological implementations—wearable fNIRS and hyperscanning—which have led to a recent surge in more ecologically valid, inclusive, and diverse research. 2.1.Global fNIRS“Global fNIRS” has become a catch-all term to describe the use of fNIRS in contexts that are underrepresented in the field of developmental neuroscience and cognition, in particular within low- and middle-income countries, low financial/health/education resource settings, and/or rural/hard-to-reach communities (for a recent review of the inclusivity of developmental neuroimaging studies, see Ref. 6). The majority of research on children’s cognitive development conducted in global health contexts had been limited to behavioral assessments to measure the effect of exposure to early adversity or strengths-based investigations of cognition. Such measures are often undertaken later in childhood rather than at the time that vulnerability to exposure is most critical (i.e., during prenatal and early postnatal life26). Beginning with a study published in 2014, by researchers working in The Gambia to understand the deleterious impact of undernutrition on brain development,27 fNIRS has begun to be increasingly used in “global” contexts. The majority of these studies are embedded within larger-scale global health studies, which has meant that the technique is also increasingly applied in longitudinal (0 to 5/7 years) and/or multiple-age-group (5 to 15 time points) experimental designs.27–32 Further, in a new clinical efficacy trial of nutritional intervention in The Gambia (Improving Infant Neurocognitive Development and Growth Outcomes with Micronutrients Trial; 10.1186/ISRCTN15063705), fNIRS will be applied longitudinally in a sample of 600 infants, which, to our knowledge, will be the largest fNIRS developmental study to date. By integrating fNIRS into these multimeasure, large-scale studies, researchers hope to better understand early trajectories of brain development. The aims are to both understand the deleterious impacts of adversity, such as psychosocial and environmental risk, and the potential beneficial impact of factors, such as enriched resource family context (i.e., multiple caregivers and supportive community practices). To better represent our understanding of child development by diversifying populations under study,33 we must create better pathways for access to neuroimaging for Majority World countries (those outside of G8 countries, where 85% of the world’s population live but currently only 5% to 15% of child developmental research has been conducted33–35), including reducing the cost and accessibility of technology that to date has generally been developed and sold in Minority World countries (such as Europe, United States Canada, Australia, and Japan). 2.2.Advances in Wearable fNIRS TechnologyWearable or mobile fNIRS refers to miniaturized wireless devices that are embedded within the headgear or other apparel that the participant wears (Fig. 2) and has been widely used in adults, and increasingly used in developmental populations.37,38 This is in contrast to more conventional table-top/trolley fNIRS devices that are relatively heavy and large in size. Moving toward, more wearable devices have numerous scientific benefits. First, wearable devices further increase the robustness of fNIRS to participant motion facilitating investigations of naturalistic activities, such as walking and crawling. Wearable neuroimaging devices may also be well placed for answering educational questions in nursery and school settings.39 Relatedly, wearable fNIRS will likely allow longer periods of measurement, which is particularly important in clinical settings, such as the monitoring of premature infants within neonatal intensive care. As development is inherently dynamic, documenting developmental change as it unfolds, e.g., learning, recovery from trauma, or improvement due to rehabilitation, rather than detecting its effects a posteriori will allow us to better understand the mechanisms at stake, and leverage them for more efficient interventions. Wearable fNIRS will also allow hard-to-reach developmental populations to be better studied, such as individuals with mental health challenges (see Sec. 3.1), individuals who may consent to partake in community-based research but are not comfortable attending more formal institutes and those living in lower income and Majority World countries (see Sec. 2.1). Finally, due to the miniaturization of hardware, concurrent recording of other physiological measures, such as electrocardiography, electromyography (EMG), and EEG is now far more achievable (Fig. 2). Fig. 2Families taking part in studies using wearable fNIRS systems: (a) at a research lab visit (LUMO, Gowerlabs) and (b) during a home visit as part of the longitudinal PIPKIN Study,36 (c) in a lab study on language development (NIRSport 2, NIRx), while (d) hyperscanning mother and child (Brite MKII, Artinis) combined with a wireless EMG system (pico EMG, Cometa; at the outer corner of the eyes) to measure facial muscle activity, and (e) while freely locomoting inside a building (PHOTON, Cortivision). ![]() Although the movement to wearables already has a number of scientific benefits, technological improvement is still ongoing, e.g., by increasing crucial factors, such as the sampling and coverage of the cortex. For example, the technological advancement of diffuse optical tomography within wearable hardware has increased signal-to-noise ratios, coupled with reduced exclusion rates in human infants.40 Thus the cutting edge of fNIRS systems in terms of the density and quality of recordings are emerging together with the move toward wearable, infant-friendly fNIRS systems. 2.3.HyperscanningSimilarly supported by the relative physical ease of recording, fNIRS neuroimaging during a live social interaction or hyperscanning has been growing rapidly in the last decade and a literature focusing on hyperscanning of infants and caregivers is emerging [Fig. 2(d)]. Hyperscanning studies with adults have provided significant knowledge in social neuroscience.20 Although there are currently a small number of studies on fNIRS hyperscanning between older children or between an adult and an older child,41–44 those with infants are more limited.45 For example, a preliminary study20 measuring mother–infant synchronization while mothers were holding their 2- to 3-month-old infants indicated stronger interpersonal synchronization of the anterior left OFC during the holding condition (versus the separation condition). Similarly, most hyperscanning studies with young populations have reported higher interpersonal coherence during cooperation or interactive behavior. These studies established a fundamental initial step in this method. However, some crucial issues require further efforts. First, precise behavioral coding of social signals (e.g., eye contact) including microsignals, preferably employing AI-based automatic coding, should be associated with fNIRS data to better establish mechanisms of synchronization.46 To fully understand the signals obtained, analysis methods, such as transfer entropy and mutual information, assessing causal relationships and amount of information exchange between data from multiple participants needs to be better established. Currently, published data most often center on global patterns of synchronization across all channels. The full potential of the spatial resolution of fNIRS should be utilized to investigate region-specific synchronization to identify more precise brain networks at play, and how these change over development. Finally, as a common approach for hyperscanning designs is to use them in more interactive “in the wild” studies, standardized methods for denoising and the removal of motion or systemic artifacts should be established, as natural interaction is accompanied with a dense and divers range of body movements and systemic signals. Predefining the frequency range for measures of synchronization, such as wavelet transformed coherence, will contribute to solving this issue. 3.Individual Variation: Uncovering Different Developmental TrajectoriesMost traditional developmental studies investigate phenomena at the level of a group of participants. There is, however, increasing interest in individual variation and the diversity of developmental trajectories, coupled with a need to understand why these differences exist. For developmental fNIRS research to better assess individual variation, we need to elucidate differences in the aetiology of vascular and metabolic pathways within specific and more general populations. At least two new directions have addressed these challenges: large-scale longitudinal studies (such as some global fNIRS studies) and research focusing on individuals with a broad range of developmental trajectories (e.g., infants born prematurely, children with developmental disorders, difficulties with hearing, or vision, etc.; for review see Ref. 47). 3.1.Developmental DisordersSome children with developmental disorders may find an fNIRS study easier to participate in than an fMRI study due to challenges associated with their condition [e.g., co-occurrence of anxiety or aversion to loud noises and confined spaces for some individuals with autism spectrum disorder (ASD/ADHD)]. Indeed, fNIRS has been successfully used in several developmental disorders, identifying altered brain function, anatomy, or connectivity in individuals with developmental disorders, e.g., in autism spectrum disorder,48,49 including nonverbal populations,50 to study social cognition, language, and executive function. Furthermore, several studies51–54 have reported different patterns of cortical responses to social signals in infants at elevated familial likelihood of developing ASD around 5 months of age, relative to typical likelihood infants. Importantly, in one study, infant responses early in development correlated with traits of ASD at 3 years of age.53 In addition to evidencing the development of the social network in ASD, these studies also indicated the feasibility of the fNIRS signal as a neural marker to detect ASD. These findings have highlighted a potential strength for the use of fNIRS in populations where different patterns of brain development may be evident at an earlier age than behavioral changes, and thus could help target family support and interventions for those infants and families in need. 3.2.Assessing Clinical InterventionsfNIRS has also been applied to study individual variation in clinical interventions or treatment. It is particularly well suited to image infants with cochlear implants, as other imaging modalities are not compatible with the device (fMRI due to the metallic parts and EEG due to signal interference). In infants with severe hearing impairment who experience auditory deprivation and the subsequent improvement of hearing following cochlear implantation, fNIRS has great potential to improve therapeutic outcomes and further our understanding of language development.55,56 Indeed, one study observed that the amplitude of the fNIRS response to human voice versus nonvoice stimuli in hard-of-hearing infants at 12 months was correlated with their residual hearing.55 3.3.Preterm Birth and Perinatal TransitionsA further clinical area of interest for studying individual variation is preterm birth as this has been associated with risks for cognitive impairments, including language delay and ADHD. In one such study,57 infants born prematurely ( weeks gestation) were tested in an audiovisual learning and prediction task. The authors found that while the neural and behavioral signatures of audiovisual learning were intact, infants did not show neural signatures of sensory prediction (also see Refs. 58 and 59). The study of individual variation, in particular within premature infants, highlights an opportunity for study but also a challenge for fNIRS research. In particular, the metabolic and synaptic systems of the brain are rapidly developing during the prenatal and early postnatal periods. Perinatal fNIRS of preterm and term neonates has provided insights into cortical angiogenesis and neurogenesis. A series of studies with this population highlighted that neural responses measured by EEG and hemodynamic responses measured by NIRS can be decoupled in premature newborns.60–62 Under some conditions, close-to-typical EEG responses were associated with weak NIRS responses and reduced blood flow, suggesting that neurovascular coupling is immature and highlighting the risk that when active, neurons may remain without the necessary metabolic nutrients in premature neonates. Furthermore, the Hb phase of oxygenation and deoxygenation, referred to as hPod,63 showed changes from an in-phase to an antiphase pattern according to postnatal age (PNA), but early preterm infants showed slower changes. Although this Hb phase development gradually continues at least until 10 months of age,64,65 its relationship with cognitive function remained unclear. Examining resting-state connectivity in preterm and term neonates, one study66 suggested that how perinatal neurovascular and metabolic systems relate to neurocognitive networks may be captured by the long-range connectivity. In contrast to the canonical hemodynamic response, characterized by an increase in oxy-Hb with a slight decrease in deoxy-Hb, younger infants tend to show variable, or sometimes opposing patterns of activation, which can be mediated by gestational and PNA, stimulus quality, or task difficulty.67–69 Vascular changes over development pose a particular challenge for longitudinal work and for drawing comparisons across populations/ages. To assess individual variation in more depth, future work should place more focus on larger cross-sectional samples and/or longitudinal frameworks to track age-related changes, taking contemporaneous measurements of brain function and behavior in parallel with measurements of the environment, such as adversity, health, community, and family context to further understand why differences exist. 4.Statistical and Analytic AdvancesAlthough the previous sections have noted a number of technical, methodological, and analytic challenges, the field of infant fNIRS (and fNIRS as a whole) has been making substantial progress on these fronts. Here we outline a selection of recent statistical and analytic advances that promise to further the field. 4.1.Resting-State NetworksThe resting-state network (RSN) is the measurement of networks in the absence of sensory or cognitive constraints, tasks, or stimulation. These networks are principally based on structurally connected regions, reflect coordinated functional networks, such as default-mode networks and executive control networks, and have widely been studied with fNIRS (as well as fMRI). To summarize the current findings, there appears to be a shift from stronger short-range to long-range connectivity across infancy but some connections show U-shaped development.66,70–72 Importantly, state of alertness (sleep/wakefulness) also affects the RSN.71,73 Several analysis methods have been proposed and utilized in fNIRS, including a general linear model (GLM)-based analysis, dynamic-causal modeling,74 independent component analysis,75 joint probability distribution of phase,76 and dynamic functional connectivity77 to establish resting state connectivity. Directionality of the connectivity can be assessed using methods, such as directional phase transfer entropy and directional connectivity. In infant fNIRS studies, a popular method is GLM-based analysis mostly using the cross-correlation of Hb changes. The phase-based analysis using instantaneous phase is also a useful method for assessing functional connectivity from within averaged block design data as well as long-term measurement of more standard data of longer duration.69 One challenging issue with infant RSN is measurement during awake states. Awake RSN without any stimulus is feasible with very young infants (e.g., neonates), but as infants age, they require some form of stimulation to prevent them from becoming inattentive and restless. Visual stimulation that catches infants’ attention has been used72 but has limitations with regards to a full understanding of what is being captured relative to true RSN paradigms. Overall, a standard set of approaches would be beneficial to allow across-lab data collection. Unlike fMRI, fNIRS data benefits from the measurement of both oxy-Hb and deoxy-Hb, and therefore, could provide insights into RSNs, yet to-date some studies report only oxy-Hb. NIRS even allows for additional measures to be used in identifying RSN, e.g., total-Hb78 and cytochrome-C-oxidase (oxCCO).54 It will be highly important to understand how we can effectively utilize all four measures of hemoglobin and oxCCO for connectivity analysis, including frequency-dependent connectivity. 4.2.Task-Based Functional ConnectivityComplementing the resting-state connectivity approaches, there are emerging analytic techniques for understanding neural networks that infants employ while they are engaged cognitively. Here we refer to this technique as task-based functional connectivity. For example, a recent study79 found a large frontoparietal network in young infants that was engaged when infants were participating in a sequence prediction task (versus perceptual control task). Importantly, this differential functional engagement was found within a single experimental session showing dynamic engagement according to an infant’s cognitive state. This technique holds promise to expand our understanding of the neural mechanisms supporting infant cognition and how they change with development from investigating activity of individual regions to how regions collectively engage and form large-scale networks. However, the relevant analytic procedures are still being developed in infant fNIRS with many decision points needed to be mapped out (see Ref. 80 for a systematic investigation of some of these decision points). How functional networks change in time, not only throughout development, but also dynamically during a task is of great relevance. This could be investigated on a trial-by-trial basis or for a longer period of time (e.g., over 5 to 8 consecutive blocks79), over an uninterrupted timecourse. 4.3.Machine Learning and Decoding ApproachesMachine learning and decoding approaches have a number of benefits including allowing researchers to look at patterns of activity across channels and using data-driven versus hypothesis-testing approaches. In general, a subset of the data (either a subset for each infant or a subset of the infants in the sample) is used to create or train a computer model and then this model is used to analyze or “decode” the remaining data. A recent study81 found evidence that infant data can be readily decoded using simple machine learning techniques and presented a task where univariate analyses (i.e., each channel analyzed separately) would not have revealed differential neural activation while decoding approaches did. As the types of machine learning techniques applied to neural data grows, so too do the possibilities for this technique. For example, explainable artificial intelligence models were used82 to reveal new relationships between patterns of activation in a previously analyzed dataset. Although there is a lot of promise in this approach, there are a number of challenges. First, many machine learning approaches need a large amount of data to train elaborate computer models, which is challenging for infancy researchers. Also the statistical tests are not as clear-cut with these techniques, and traditional power analyses are not applicable (see Ref. 81 for bootstrapped statistical tests). Finally, it is important to think clearly about what scientific questions these techniques can answer and how they are similar or different from more traditional-hypothesis testing techniques. With a growing complement of analytic approaches, the linking hypothesis between question and test is ever more important. 4.4.Reproducibility, Meta-Analyses, and Collaborative Replication ProjectsConcerns about reproducibility have recently emerged in many areas of empirical research from psychology and sociology to neuroscience and medicine, as some key findings were found not to replicate reliably.83–86 A number of the factors that have led to the replicability crisis are particularly serious in developmental research, as young children are challenging participants, which may lead to large amounts of missing data, imprecise data rejection criteria, short study durations, small numbers of test trials, small sample sizes, and relatedly, low statistical power. Developmental fNIRS research is no exception. There is thus a clear need in the developmental fNIRS community to address issues of replicability. A number of complementary solutions are emerging to address this issue. One approach is to conduct a meta-analysis of existing published and/or unpublished studies that address the same research question. A meta-analysis is a quantitative method for gathering data from experimental studies into a larger dataset in order to calculate the mean effect size of a specific phenomenon and assess its variation across studies.87 Additionally, the contribution of different moderators (i.e., factors accounting for cross-study variation, such as type of NIRS machine used or age of participants) may be statistically evaluated and biases in the published literature like the “file drawer effect” (i.e., failure to publish null results) may be identified. Meta-analyses have been successfully conducted over behavioral data in developmental populations.88,89 To date, very few studies have carried out meta-analyses on developmental fNIRS data.5,90,91 These studies suggest that at least some of the published fNIRS results are robust to replication and have small-to-moderate effect sizes, which is typical of developmental studies conducted with other methods.88 Further studies will be needed in the future to systematically assess the replicability and effect sizes of key findings in the developmental fNIRS literature. Collaborative, multilaboratory studies, whereby a given experimental protocol is carried out in a large number of different labs using methodologies as similar as possible is another approach to address the replicability crisis. Such studies, with their increased sample sizes, can provide higher statistical power and more robust conclusions than single studies. Such collaborative studies have successfully been conducted in many areas of general83 and developmental92 psychology. Currently, a collaborative, multilab replication project is ongoing aiming to address the cross-lab variability of young infants’ rule-learning ability in language as indexed by fNIRS. This ManyBabies 3 NIRS project93 (Fig. 3; https://manybabies.github.io/MB3N/) brings together 35 laboratories from 11 countries across 4 continents. The preparation and harmonization of research protocols is ongoing, and the resulting methods will be submitted as a registered report. 5.DiscussionThe use of fNIRS to image the developing brain has grown considerably since its first applications nearly two and a half decades ago. Technological development and methodological innovations have allowed researchers to obtain increasingly high-quality data in the lab even from the very challenging populations of infants and toddlers. As we look into the future of developmental fNIRS, the next challenge is to take fNIRS studies out of the laboratories and into the wild. Moving away from the laboratory will allow the developmental fNIRS community to obtain a more accurate, less biased understanding of human neural development and to test participants while they are performing behaviors or are placed in settings that correspond more closely to real-life situations. Although these initiatives correspond to more general trends in psychology and neuroscience,94 fNIRS is particularly well-suited to achieve these goals. One consequence of moving research to more naturalistic and global settings is that researchers will have less control over the environmental variables, such as illumination, temperature, ambient noise, and distractors in the environment, that might influence infants’ behavior. As a consequence, variability in the data may increase (though see Ref. 95), making a focus on power and analytic techniques all the more important. Initiatives to conduct fNIRS in the wild go hand-in-hand with efforts to quantify variability, assess replicability, and increase sample sizes, again following more general tendencies in research with human participants.86 Indeed, standardization has been and will likely continue to be, a major goal in the field,96 and new data analysis techniques, statistical methods, and replication projects are emerging. Overall, we see these advances and the new challenges in developmental fNIRS as an opportunity to analyze the brain as a multiscale system operating in a highly complex environment. Complementing more traditional localization-based approaches in single populations and age groups studied in a single lab with fNIRS as the only or main dependent measure, the field is moving toward the analysis of multimodal datasets where fNIRS data need to be combined with data from other imaging, physiological or behavioral measures. Furthermore, there is a drive to provide access to more standardized and openly communicated research procedures as well as to establish research hubs and networks that span multiple international sites. Developmental fNIRS is moving toward collecting data with numerous diverse populations giving us a more complex and complete picture of human neural development. 6.ConclusionWe see the advances and the challenges in developmental fNIRS discussed above as an opportunity to analyze the brain as a multiscale system operating in a highly complex environment. Complementing more traditional localization-based approaches in single populations and age groups studied in a single lab with fNIRS as the only or main dependent measure, the field is moving toward the analysis of multimodal datasets where fNIRS data need to be combined with data from other imaging, physiological or behavioral measures. Furthermore, there is a drive to provide access to more standardized and openly communicated research procedures as well as to establish research hubs and networks that span multiple international sites. Developmental fNIRS is moving toward collecting data with numerous diverse populations giving us a more complex and complete picture of human neural development. DisclosuresThe authors declare no relevant financial interests or other potential conflicts of interest. AcknowledgmentsThis work was funded by the ERC Consolidator Grant “BabyRhythm” (Grant No. 773202) to J.G., a UKRI Future Leaders fellowship (Grant No. MR/S018425/1) and Bill & Melinda Gates Foundation (BMGF) (Grant No. OPP1127625) to S.L.F., a MEXT Grants-in-Aid for Scientific Research (S) (Grant No. 19H05594) to Y.M., and NSERC Discovery Grant and McDonnell Foundation (Grant No. AWD1005451) to L.L.E. The views expressed are those of the authors and not necessarily those of the ERC, UKRI. MEXT, NSERC, the BMGF, or the McDonnell Foundation. ReferencesJ. H. Meek et al.,
“Regional hemodynamic responses to visual stimulation in awake infants,”
Pediatr. Res., 43
(6), 840
–843 https://doi.org/10.1203/00006450-199806000-00019 PEREBL 0031-3998
(1998).
Google Scholar
S. Lloyd-Fox, A. Blasi and C. E. Elwell,
“Illuminating the developing brain: the past, present and future of functional near infrared spectroscopy,”
Neurosci. Biobehav. Rev., 4 269
–284 https://doi.org/10.1016/j.neubiorev.2009.07.008 NBREDE 0149-7634
(2009).
Google Scholar
J. Gervain et al.,
“Near-infrared spectroscopy: a report from the McDonnell infant methodology consortium,”
Dev. Cognit. Neurosci., 1
(1), 22
–46 https://doi.org/10.1016/j.dcn.2010.07.004
(2011).
Google Scholar
R. N. Aslin,
“Questioning the questions that have been asked about the infant brain using near-infrared spectroscopy,”
Cognit. Neuropsychol., 29
(1–2), 7
–33 https://doi.org/10.1080/02643294.2012.654773 COGNEP 1464-0627
(2012).
Google Scholar
S. Baek et al.,
“Attrition rate in infant fNIRS research: a meta-analysis,”
Infancy, https://doi.org/10.1111/infa.12521
(2023).
Google Scholar
A. Azhari et al.,
“A decade of infant neuroimaging research: what have we learned and where are we going?,”
Infant Behav. Dev., 58 101389 https://doi.org/10.1016/j.infbeh.2019.101389 IBDEDP
(2020).
Google Scholar
R. N. Aslin, M. Shukla and L. L. Emberson,
“Hemodynamic correlates of cognition in human infants,”
Annu. Rev. Psychol., 66 349 https://doi.org/10.1146/annurev-psych-010213-115108 ARPSAC 0066-4308
(2015).
Google Scholar
Y. Minagawa-Kawai et al.,
“Optical imaging of infants’ neurocognitive development: recent advances and perspectives,”
Dev. Neurobiol., 68
(6), 712
–728 https://doi.org/10.1002/dneu.20618
(2008).
Google Scholar
L. Kaplan, B. W. Chow and C. Gu,
“Neuronal regulation of the blood–brain barrier and neurovascular coupling,”
Nat. Rev. Neurosci., 21
(8), 416
–432 https://doi.org/10.1038/s41583-020-0322-2 NRNAAN 1471-003X
(2020).
Google Scholar
C. Iadecola,
“The neurovascular unit coming of age: a journey through neurovascular coupling in health and disease,”
Neuron, 96
(1), 17
–42 https://doi.org/10.1016/j.neuron.2017.07.030 NERNET 0896-6273
(2017).
Google Scholar
M. Matsui et al.,
“Referential framework for transcranial anatomical correspondence for fNIRS based on manually traced sulci and gyri of an infant brain,”
Neurosci. Res., 80 55
–68 https://doi.org/10.1016/j.neures.2014.01.003
(2014).
Google Scholar
L. H. Collins-Jones et al.,
“Longitudinal infant fNIRS channel-space analyses are robust to variability parameters at the group-level: an image reconstruction investigation,”
NeuroImage, 237 118068 https://doi.org/10.1016/j.neuroimage.2021.118068 NEIMEF 1053-8119
(2021).
Google Scholar
T. Wilcox et al.,
“Hemodynamic response to featural changes in the occipital and inferior temporal cortex in infants: a preliminary methodological exploration,”
Dev. Sci., 11
(3), 361
–370 https://doi.org/10.1111/j.1467-7687.2008.00681.x
(2008).
Google Scholar
S. Shimada and K. Hiraki,
“Infant’s brain responses to live and televised action,”
Neuroimage, 32
(2), 930
–939 https://doi.org/10.1016/j.neuroimage.2006.03.044 NEIMEF 1053-8119
(2006).
Google Scholar
S. Lloyd-Fox et al.,
“Are you talking to me? Neural activations in 6-month-old infants in response to being addressed during natural interactions,”
Cortex, 70 35
–48 https://doi.org/10.1016/j.cortex.2015.02.005
(2015).
Google Scholar
A. A. Baird et al.,
“Frontal lobe activation during object permanence: data from near-infrared spectroscopy,”
NeuroImage, 16
(4), 1120
–1126 https://doi.org/10.1006/nimg.2002.1170 NEIMEF 1053-8119
(2002).
Google Scholar
N. M. McDonald and K. L. Perdue,
“The infant brain in the social world: moving toward interactive social neuroscience with functional near-infrared spectroscopy,”
Neurosci. Biobehav. Rev., 87 38
–49 https://doi.org/10.1016/j.neubiorev.2018.01.007 NBREDE 0149-7634
(2018).
Google Scholar
S. Urakawa et al.,
“Selective medial prefrontal cortex responses during live mutual gaze interactions in human infants: an fNIRS study,”
Brain Topogr., 28
(5), 691
–701 https://doi.org/10.1007/s10548-014-0414-2 BRTOEZ 0896-0267
(2015).
Google Scholar
M. Xu et al.,
“Prefrontal function engaging in external-focused attention in 5-to 6-month-old infants: a suggestion for default mode network,”
Front. Hum. Neurosci., 10 676 https://doi.org/10.3389/fnhum.2016.00676
(2017).
Google Scholar
Y. Minagawa, M. Xu and S. Morimoto,
“Toward interactive social neuroscience: neuroimaging real-world interactions in various populations,”
Jpn. Psychol. Res., 60
(4), 196
–224 https://doi.org/10.1111/jpr.12207
(2018).
Google Scholar
B. Bonevski et al.,
“Reaching the hard-to-reach: a systematic review of strategies for improving health and medical research with socially disadvantaged groups,”
BMC Med. Res. Methodol., 14
(1), 42 https://doi.org/10.1186/1471-2288-14-42
(2014).
Google Scholar
M. M. Arredondo,
“Shining a light on cultural neuroscience: recommendations on the use of fNIRS to study how sociocultural contexts shape the brain,”
Cultur. Divers. Ethnic Minor. Psychol., 29 106
–117 https://doi.org/10.1037/cdp0000469
(2021).
Google Scholar
A. Blasi et al.,
“fNIRS for tracking brain development in the context of global health projects,”
Photonics, 6
(3), 89 https://doi.org/10.3390/photonics6030089
(2019).
Google Scholar
K. K. Jasińska and S. Guei,
“Neuroimaging field methods using functional Near-Infrared Spectroscopy (NIRS) neuroimaging to study global child development: rural sub-Saharan Africa,”
J. Vis. Exp., 132 57165 https://doi.org/10.3791/57165
(2018).
Google Scholar
A. K. Fishell et al.,
“Portable, field-based neuroimaging using high-density diffuse optical tomography,”
NeuroImage, 215 116541 https://doi.org/10.1016/j.neuroimage.2020.116541 NEIMEF 1053-8119
(2020).
Google Scholar
S. Sabanathan, B. Wills and M. Gladstone,
“Child development assessment tools in low-income and middle-income countries: how can we use them more appropriately?,”
Arch. Dis. Child., 100
(5), 482
–488 https://doi.org/10.1136/archdischild-2014-308114 ADCHAK 0003-9888
(2015).
Google Scholar
S. Lloyd-Fox et al.,
“Coregistering functional near-infrared spectroscopy with underlying cortical areas in infants,”
Neurophotonics, 1
(2), 025006 https://doi.org/10.1117/1.NPh.1.2.025006
(2014).
Google Scholar
S. Lloyd-Fox et al.,
“Cortical specialisation to social stimuli from the first days to the second year of life: a rural Gambian cohort,”
Dev. Cognit. Neurosci., 25 92
–104 https://doi.org/10.1016/j.dcn.2016.11.005
(2017).
Google Scholar
K. L. Perdue et al.,
“Using functional near-infrared spectroscopy to assess social information processing in poor urban Bangladeshi infants and toddlers,”
Dev. Sci., 22
(5), e12839 https://doi.org/10.1111/desc.12839
(2019).
Google Scholar
L. Pirazzoli et al.,
“Association of psychosocial adversity and social information processing in children raised in a low-resource setting: an fNIRS study,”
Dev. Cognit. Neurosci., 56 101125 https://doi.org/10.1016/j.dcn.2022.101125
(2022).
Google Scholar
S. Wijeakumar et al.,
“Early adversity in rural India impacts the brain networks underlying visual working memory,”
Dev. Sci., 22
(5), e12822 https://doi.org/10.1111/desc.12822
(2019).
Google Scholar
S. Lloyd-Fox et al.,
“Habituation and novelty detection fNIRS brain responses in 5-and 8-month-old infants: the Gambia and UK,”
Dev. Sci., 22
(5), e12817 https://doi.org/10.1111/desc.12817
(2019).
Google Scholar
C. E. Draper et al.,
“Publishing child development research from around the world: an unfair playing field resulting in most of the world’s child population under-represented in research,”
Infant Child Dev., e2375 https://doi.org/10.1002/icd.2375
(2022).
Google Scholar
M. Nielsen et al.,
“The persistent sampling bias in developmental psychology: a call to action,”
J. Exp. Child Psychol., 162 31
–38 https://doi.org/10.1016/j.jecp.2017.04.017 JECPAE 0022-0965
(2017).
Google Scholar
Y. Moriguchi,
“Beyond bias to Western participants, authors, and editors in developmental science,”
Infant Child Dev., 31
(1), e2256 https://doi.org/10.1002/icd.2256
(2022).
Google Scholar
P. Pinti et al.,
“A review on the use of wearable functional Near-Infrared Spectroscopy in naturalistic environments,”
Jpn. Psychol. Res., 60
(4), 347
–373 https://doi.org/10.1111/jpr.12206
(2018).
Google Scholar
A. von Lühmann et al.,
“Toward Neuroscience of the Everyday World (NEW) using functional Near-Infrared Spectroscopy,”
Curr. Opin. Biomed. Eng., 18 100272 https://doi.org/10.1016/j.cobme.2021.100272
(2021).
Google Scholar
T. W. P. Janssen et al.,
“Opportunities and limitations of mobile neuroimaging technologies in educational neuroscience,”
Mind Brain Educ., 15
(4), 354
–370 https://doi.org/10.1111/mbe.12302
(2021).
Google Scholar
E. M. Frijia et al.,
“Functional imaging of the developing brain with wearable high-density diffuse optical tomography: a new benchmark for infant neuroimaging outside the scanner environment,”
NeuroImage, 225 117490 https://doi.org/10.1016/j.neuroimage.2020.117490 NEIMEF 1053-8119
(2021).
Google Scholar
V. Reindl et al.,
“Brain-to-brain synchrony in parent-child dyads and the relationship with emotion regulation revealed by fNIRS-based hyperscanning,”
NeuroImage, 178 493
–502 https://doi.org/10.1016/j.neuroimage.2018.05.060 NEIMEF 1053-8119
(2018).
Google Scholar
T. Nguyen et al.,
“The effects of interaction quality on neural synchrony during mother-child problem solving,”
Cortex, 124 235
–249 https://doi.org/10.1016/j.cortex.2019.11.020
(2020).
Google Scholar
J. G. Miller et al.,
“Inter-brain synchrony in mother-child dyads during cooperation: an fNIRS hyperscanning study,”
Neuropsychologia, 124 117
–124 https://doi.org/10.1016/j.neuropsychologia.2018.12.021 NUPSA6 0028-3932
(2019).
Google Scholar
A. Azhari, A. Bizzego and G. Esposito,
“Father-child dyads exhibit unique inter-subject synchronization during co-viewing of animation video stimuli,”
Soc. Neurosci., 16
(5), 522
–533 https://doi.org/10.1080/17470919.2021.1970016
(2021).
Google Scholar
T. Nguyen et al.,
“Proximity and touch are associated with neural but not physiological synchrony in naturalistic mother-infant interactions,”
NeuroImage, 244 118599 https://doi.org/10.1016/j.neuroimage.2021.118599 NEIMEF 1053-8119
(2021).
Google Scholar
M. Xu et al.,
“Two-in-one system and behavior-specific brain synchrony during goal-free cooperative creation: an analytical approach combining automated behavioral classification and the event-related generalized linear model,”
Neurophotonics, 10
(1), 013511 https://doi.org/10.1117/1.NPh.10.1.013511
(2023).
Google Scholar
R. E. Vanderwert and C. A. Nelson,
“The use of near-infrared spectroscopy in the study of typical and atypical development,”
Neuroimage, 85 264
–271 https://doi.org/10.1016/j.neuroimage.2013.10.009 NEIMEF 1053-8119
(2014).
Google Scholar
L. E. Libero et al.,
“Multimodal neuroimaging based classification of autism spectrum disorder using anatomical, neurochemical, and white matter correlates,”
Cortex, 66 46
–59 https://doi.org/10.1016/j.cortex.2015.02.008
(2015).
Google Scholar
S. Sutoko et al.,
“Distinct methylphenidate-evoked response measured using functional near-infrared spectroscopy during Go/No-Go task as a supporting differential diagnostic tool between attention-deficit/hyperactivity disorder and autism spectrum disorder comorbid children,”
Front. Hum. Neurosci., 13 7 https://doi.org/10.3389/fnhum.2019.00007
(2019).
Google Scholar
Y. Minagawa-Kawai et al.,
“Cerebral laterality for phonemic and prosodic cue decoding in children with autism,”
NeuroReport, 20
(13), 1219
–1224 https://doi.org/10.1097/WNR.0b013e32832fa65f NERPEZ 0959-4965
(2009).
Google Scholar
R. Braukmann et al.,
“Diminished socially selective neural processing in 5-month-old infants at high familial risk of autism,”
Eur. J. Neurosci., 47
(6), 720
–728 https://doi.org/10.1111/ejn.13751 EJONEI 0953-816X
(2018).
Google Scholar
S. Lloyd-Fox et al.,
“Reduced neural sensitivity to social stimuli in infants at risk for autism,”
Proc. R. Soc. B Biol. Sci., 280
(1758), 20123026 https://doi.org/10.1098/rspb.2012.3026
(2013).
Google Scholar
S. Lloyd-Fox et al.,
“Cortical responses before 6 months of life associate with later autism,”
Eur. J. Neurosci., 47
(6), 736
–749 https://doi.org/10.1111/ejn.13757 EJONEI 0953-816X
(2018).
Google Scholar
M. F. Siddiqui et al.,
“Non-invasive measurement of a metabolic marker of infant brain function,”
Sci. Rep., 7 1330 https://doi.org/10.1038/s41598-017-01394-z SRCEC3 2045-2322
(2017).
Google Scholar
A. Coëz et al.,
“The recognition of human voice in deaf and hearing infants,”
Hear. Balance Commun., 20
(3), 179
–185 https://doi.org/10.1080/21695717.2022.2084866
(2022).
Google Scholar
H. Bortfeld,
“Functional near-infrared spectroscopy as a tool for assessing speech and spoken language processing in pediatric and adult cochlear implant users,”
Dev. Psychobiol., 61
(3), 430
–443 https://doi.org/10.1002/dev.21818 DEPBA5 0012-1630
(2019).
Google Scholar
L. L. Emberson et al.,
“Deficits in top–down sensory prediction in infants at risk due to premature birth,”
Curr. Biol., 27
(3), 431
–436 https://doi.org/10.1016/j.cub.2016.12.028 CUBLE2 0960-9822
(2017).
Google Scholar
S. Jaffe-Dax et al.,
“A computational role for top–down modulation from frontal cortex in infancy,”
J. Cognit. Neurosci., 32
(3), 508
–514 https://doi.org/10.1162/jocn_a_01497 JCONEO 0898-929X
(2020).
Google Scholar
A. M. Boldin, R. Geiger and L. L. Emberson,
“The emergence of top–down, sensory prediction during learning in infancy: a comparison of full-term and preterm infants,”
Dev. Psychobiol., 60
(5), 544
–556 https://doi.org/10.1002/dev.21624 DEPBA5 0012-1630
(2018).
Google Scholar
M. Mahmoudzadeh et al.,
“Syllabic discrimination in premature human infants prior to complete formation of cortical layers,”
Proc. Natl. Acad. Sci. U. S. A., 110
(12), 4846
–4851 https://doi.org/10.1073/pnas.1212220110
(2013).
Google Scholar
M. Nourhashemi et al.,
“Relationship between relative cerebral blood flow, relative cerebral blood volume, and relative cerebral metabolic rate of oxygen in the preterm neonatal brain,”
Neurophotonics, 4
(2), 021104 https://doi.org/10.1117/1.NPh.4.2.021104
(2017).
Google Scholar
F. Wallois et al.,
“Back to basics: the neuronal substrates and mechanisms that underlie the electroencephalogram in premature neonates,”
Neurophysiol. Clin., 51
(1), 5
–33 https://doi.org/10.1016/j.neucli.2020.10.006 NCLIE4 0987-7053
(2021).
Google Scholar
H. Watanabe et al.,
“Hemoglobin phase of oxygenation and deoxygenation in early brain development measured using fNIRS,”
Proc. Natl. Acad. Sci. U. S. A., 114
(9), E1737
–E1744 https://doi.org/10.1073/pnas.1616866114
(2017).
Google Scholar
G. Taga, H. Watanabe and F. Homae,
“Spatial variation in the hemoglobin phase of oxygenation and deoxygenation in the developing cortex of infants,”
Neurophotonics, 5
(1), 011017 https://doi.org/10.1117/1.NPh.5.1.011017
(2017).
Google Scholar
Z. Liang et al.,
“Tracking brain development from neonates to the elderly by hemoglobin phase measurement using functional near-infrared spectroscopy,”
IEEE J. Biomed. Health Inform., 25
(7), 2497
–2509 https://doi.org/10.1109/JBHI.2021.3053900
(2021).
Google Scholar
T. Arimitsu et al.,
“Differential age-dependent development of inter-area brain connectivity in term and preterm neonates,”
Pediatr. Res., 92 1017
–1025 https://doi.org/10.1038/s41390-022-01939-7 PEREBL 0031-3998
(2022).
Google Scholar
C. Issard and J. Gervain,
“Variability of the hemodynamic response in infants: influence of experimental design and stimulus complexity,”
Dev. Cognit. Neurosci., 33 182
–193 https://doi.org/10.1016/j.dcn.2018.01.009
(2018).
Google Scholar
T. Arimitsu et al.,
“The cerebral hemodynamic response to phonetic changes of speech in preterm and term infants: the impact of postmenstrual age,”
NeuroImage Clin., 19 599
–606 https://doi.org/10.1016/j.nicl.2018.05.005
(2018).
Google Scholar
M. Uchida-Ota et al.,
“Maternal speech shapes the cerebral frontotemporal network in neonates: a hemodynamic functional connectivity study,”
Dev. Cognit. Neurosci., 39 100701 https://doi.org/10.1016/j.dcn.2019.100701
(2019).
Google Scholar
F. Homae et al.,
“Development of global cortical networks in early infancy,”
J. Neurosci., 30
(14), 4877
–4882 https://doi.org/10.1523/JNEUROSCI.5618-09.2010 JNRSDS 0270-6474
(2010).
Google Scholar
G. Taga, H. Watanabe and F. Homae,
“Developmental changes in cortical sensory processing during wakefulness and sleep,”
NeuroImage, 178 519
–530 https://doi.org/10.1016/j.neuroimage.2018.05.075 NEIMEF 1053-8119
(2018).
Google Scholar
C. Bulgarelli et al.,
“The developmental trajectory of fronto-temporoparietal connectivity as a proxy of the default mode network: a longitudinal fNIRS investigation,”
Hum. Brain Mapp., 41
(10), 2717
–2740 https://doi.org/10.1002/hbm.24974 HBRME7 1065-9471
(2020).
Google Scholar
C. W. Lee et al.,
“Sleep state modulates resting-state functional connectivity in neonates,”
Front. Neurosci., 14 347 https://doi.org/10.3389/fnins.2020.00347 1662-453X
(2020).
Google Scholar
S. Tak et al.,
“Dynamic causal modelling for functional near-infrared spectroscopy,”
Neuroimage, 111 338
–349 https://doi.org/10.1016/j.neuroimage.2015.02.035 NEIMEF 1053-8119
(2015).
Google Scholar
H. Zhang et al.,
“Functional connectivity as revealed by independent component analysis of resting-state fNIRS measurements,”
NeuroImage, 51
(3), 1150
–1161 https://doi.org/10.1016/j.neuroimage.2010.02.080 NEIMEF 1053-8119
(2010).
Google Scholar
B. Molavi et al.,
“Analyzing the resting state functional connectivity in the human language system using near infrared spectroscopy,”
Front. Hum. Neurosci., 7 921 https://doi.org/10.3389/fnhum.2013.00921
(2014).
Google Scholar
F. S. Racz et al.,
“Multifractal dynamic functional connectivity in the resting-state brain,”
Front. Physiol., 9 1704 https://doi.org/10.3389/fphys.2018.01704 FROPBK 0301-536X
(2018).
Google Scholar
B. R. White et al.,
“Wavelength censoring for spectroscopy in optical functional neuroimaging,”
Phys. Med. Biol., 66
(6), 065026 https://doi.org/10.1088/1361-6560/abd418 PHMBA7 0031-9155
(2021).
Google Scholar
S. Baek et al.,
“Temporal predictability modulates cortical activity and functional connectivity in the frontoparietal network in 6-month-old infants,”
J. Cognit. Neurosci., 34
(5), 766
–775 https://doi.org/10.1162/jocn_a_01828 JCONEO 0898-929X
(2022).
Google Scholar
S. Jaffe-Dax et al.,
“Sequence learning attenuates cortical responses in both frontal and sensory cortices in early infancy,”
Google Scholar
L. L. Emberson et al.,
“Decoding the infant mind: multivariate pattern analysis (MVPA) using fNIRS,”
PLoS One, 12
(4), e0172500 https://doi.org/10.1371/journal.pone.0172500 POLNCL 1932-6203
(2017).
Google Scholar
J. Andreu-Perez et al.,
“Explainable artificial intelligence based analysis for interpreting infant fNIRS data in developmental cognitive neuroscience,”
Commun. Biol., 4
(1), 1
–13 https://doi.org/10.1038/s42003-021-02534-y
(2021).
Google Scholar
R. A. Klein et al.,
“Investigating variation in replicability: a ‘many labs’ replication project,”
Soc. Psychol., 45
(3), 142 https://doi.org/10.1027/1864-9335/a000178
(2014).
Google Scholar
M. R. Munafò et al.,
“A manifesto for reproducible science,”
Nat. Hum. Behav., 1
(1), 0021 https://doi.org/10.1038/s41562-016-0021
(2017).
Google Scholar
B. A. Nosek, J. R. Spies and M. Motyl,
“Scientific utopia: II. Restructuring incentives and practices to promote truth over publishability,”
Perspect. Psychol. Sci., 7
(6), 615
–631 https://doi.org/10.1177/1745691612459058
(2012).
Google Scholar
Open Science Collaboration,
“Estimating the reproducibility of psychological science,”
Science, 349
(6251), aac4716 https://doi.org/10.1126/science.aac4716 SCIEAS 0036-8075
(2015).
Google Scholar
G. Cumming, Understanding The New Statistics: Effect Sizes, Confidence Intervals, and Meta-Analysis, Routledge(
(2013). Google Scholar
C. Bergmann et al.,
“Promoting replicability in developmental research through meta-analyses: insights from language acquisition research,”
Child Dev., 89
(6), 1996
–2009 https://doi.org/10.1111/cdev.13079 CHDEAW 0009-3920
(2018).
Google Scholar
M. Lewis et al.,
“A quantitative synthesis of early language acquisition using meta-analysis,”
(2016). https://doi.org/10.31234/osf.io/htsjm Google Scholar
J. Gemignani and J. Gervain,
“Brain responses to repetition-based rule-learning do not exhibit sex differences: an aggregated analysis of infant fNIRS studies,”
Google Scholar
J. Gemignani et al.,
“Reproducibility of infant fNIRS studies: a meta-analytic approach,”
Neurophotonics, 10
(2), 023518 https://doi.org/10.1117/1.NPh.10.2.023518
(2023).
Google Scholar
ManyBabies Consortium,
“Quantifying sources of variability in infancy research using the infant-directed speech preference,”
Adv. Methods Pract. Psychol. Sci., 3 24
–52 https://doi.org/10.1177/251524591990080
(2019).
Google Scholar
J. Gervain, ManyBabies3NIRS Consortium,
“ManyBabies3NIRS: a large-scale, multi-lab NIRS replication study assessing infants’ ability to extract regularities from speech,”
in fNIRS,
(2022). Google Scholar
J. M. Box-Steffensmeier et al.,
“The future of human behaviour research,”
Nat. Hum. Behav., 6
(1), 15
–24 https://doi.org/10.1038/s41562-021-01275-6
(2022).
Google Scholar
J. R. Yurkovic et al.,
“Using head-mounted eye tracking to examine visual and manual exploration during naturalistic toy play in children with and without autism spectrum disorder,”
Sci. Rep., 11 3578 https://doi.org/10.1038/s41598-021-81102-0 SRCEC3 2045-2322
(2021).
Google Scholar
M. A. Yücel et al.,
“Best practices for fNIRS publications,”
Neurophotonics, 8
(1), 012101 https://doi.org/10.1117/1.NPh.8.1.012101
(2021).
Google Scholar
BiographyJudit Gervain is a full professor in the Department of Developmental and Social Psychology at the University of Padua, Italy, and a senior researcher at the Integrative Neuroscience and Cognition Center, CNRS, University of Paris, France. Originally trained as a theoretical linguist, she holds a PhD in cognitive neuroscience from SISSA, Trieste, Italy. She is an associate editor at Neurophotonics, Developmental Science, and Cognition. Her research focuses on the perceptual, cognitive, and neural basis of early speech perception and language development, with special attention to the impact of prenatal experience with speech on language acquisition in typical and atypical development. Yasuyo Minagawa received her PhD in medicine from the University of Tokyo. She is a professor of the Department of Psychology at Keio University. Her research interests are in the infant’s development of communication skills focusing on speech perception, production, and social cognition in typical and atypical brain development. Lauren Emberson received her PhD in psychology with a specialization in perception, cognition, and development from Cornell University. She is an assistant professor in the Department of Psychology, University of British Columbia, Canada. Her research interests are in how the brain uses experience and learning to develop perceptual capacities starting in infancy. Sarah Lloyd-Fox received her PhD in developmental neuroscience from Birkbeck, University of London. She is a senior research associate in the Department of Psychology at the University of Cambridge. She is the author of more than 50 publications, including a seminal review paper on the developmental fNIRS research. She is a lead on several collaborative multidisciplinary longitudinal cohort studies that embed fNIRS within multimeasure designs to further our understanding of individual differences and developmental trajectories in the context of global health, social and family contexts, developmental disorders, adversity, and poverty. |
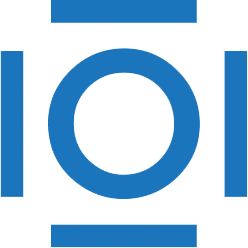
CITATIONS
Cited by 6 scholarly publications.
Brain
Near infrared spectroscopy
Neuroimaging
Machine learning
Analytical research
Neurophotonics
Statistical analysis