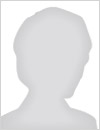
SPHERE is the VLT exo-planet imager and is based on XAO and coronagraphy. Malfunctioning DM actuators can have a severe impact on the instrument contrast. 18 dead and 8 sluggish actuators were identified during commissioning, but the actuator's behavior needs to be monitored during the whole instrument lifetime. Daily, the temporal responses of SPHERE's 1377 actuators are measured at 1380Hz. The method to automatically identify the status of the actuators is based on machine learning. We used the SciKit toolbox (INRIA, France) and implemented a Support Vector Machine algorithm. The model was trained on data acquired on 167 daily measurements of dead actuators, 73 daily measurements of sluggish actuators and 334 daily measurements of good actuators. The model was then validated on 73 daily measurements of dead actuators, 26 daily measurements of sluggish actuators and 147 daily measurements of good actuators.
The method accurately identified malfunctioning actuators with an extremely low number of false positives (1). The method is easy to implement, fast (30ms) and easily scalable to systems with more degrees of liberty such as MOEMS DMs and the future ELT DMs.Direct imaging of Earth-like exoplanets is one of the significant scientific drivers of the next generation of ground-based telescopes. Typically, Earth-like exoplanets are located at tiny angular separations from their host stars rendering their identification difficult. Consequently, the adaptive optics (AO) system’s control algorithm must be carefully designed to distinguish the exoplanet from the residual light produced by the host star.
A new promising avenue of research aimed at improving AO control builds on data-driven control methods such as Reinforcement Learning (RL) methods. It is an active branch of the machine learning research field, where control of a system is learned through interaction with the environment. Thus, RL can be seen as an automated approach for AO control. In particular, model-based reinforcement learning (MBRL) has been shown to cope with both temporal and misregistration errors. Similarly, it has been demonstrated to adapt to non-linear wavefront sensing while being efficient to train and execute.
In this work, we implement and adapt an RL method called Policy Optimizations for AO (PO4AO) to the GHOST test bench at ESO headquarters, where we show strong performance on cascaded AO system lab simulation. Further, the results align with the previously obtained results with the method.View contact details